The automotive market is going through a very important transition, which will transform this sector forever. The two most influencing trends in the automotive sector are electrification and autonomous driving, they will impact car manufacturers in many areas and will specifically have a negative effect on the aftersales business, reducing maintenance occasions. After-sales is a difficult market per se, being very mature, with an expected growth rate close to zero. In addition, competition is increasing, due to the entry of new players, which especially sell spare parts online.
All of these are important threats for car manufacturers, which risk a significant reduction of their brand’s customers who go to the official network for maintenance, instead of going to third parties repairers.

Predictive maintenance therefore represents one of the most concrete answers to these threats, aiming to foresee maintenance opportunities and consequently to retain customers, encouraging them to go to the car manufacturers’ official network.
Looking at a historical perspective, even manual inspections are predictive maintenance; in fact, very often a mechanic can decide to replace a worn out piece, foreseeing a possible breakage. This method, although effective, is very expensive, therefore, several car manufacturers have tried to automate it, by analyzing the data of previously maintained cars. To take advantage of these analyzes, many car brands have decided to install "black boxes", which suggest to customers when to go for maintenance and directly proposing appointments at the authorized network.
Ultimately, artificial intelligence may be able to make predictions in real time, even if it is not a mature technology and therefore is not yet used by any manufacturer. It can be applied to new, even more advanced, black boxes, which will further increase the perception of safety for car drivers, thanks to real-time monitoring of the vehicle's status.

To assess the actual usefulness of these tools, the OnStar case study has been analyzed. The system, installed by Opel from 2016 to 2019, is a predictive maintenance tool, based on data previously collected and analyzed, in order to suggest to customers when to go to the official Opel workshop to carry out a manual inspection of the vehicle. In addition to this, the system also offers various concierge services, but its main objective is precisely to retain more customers, improving the so-called retention rate.
To do so, the system alerts the driver when it is time to carry out maintenance and proposes appointments directly to the official network, in order to prevent the customer from going to third party repairers.

Opel has kindly provided a dataset, containing information about the country of sale of the vehicles, their age and the retention rate and average invoice, all depending on the OnStar system being installed or not. For this reason, it was possible to evaluate the impact of the system, firstly on the retention rate and secondly on the average invoice.

From the distribution analysis, the system tends to retain more customers for maintenance, thus generating incremental turnover for the car manufacturer. Splitting by country also confirms that OnStar is effective in all the nations analyzed, apart from Spain, where it is irrelevant.
Evaluating the impact on the average invoice, however, we see how it tends to decrease in case OnStar is installed. This is due to the fact people go to Opel workshops also in case of less expensive maintenances, the system indeed rises the company turnover, thanks to the higher retention rate. Finally, it can be noted that in Spain the average bill has risen, this to balance the neutral effect that the system had on the retention rate in the Iberian country.

Continuing the analysis, linear regression models were created, in order to predict both retention rate and average invoice.
First, a graphic representation of the retention rate values was given, which shows how the OnStar system is able to retain more customers after the first two years of the vehicle. This observation is very important, given that OnStar proves to be most effective right at the end of the warranty period, when maintenance is carried out by the customers and not by Opel.

The first regression model created is therefore a simple one, which predicts the retention rate based only on the years of the vehicle. The model is significant and highly explanatory; however, it was decided to proceed by adding variables, up to the complete model, which provides for the retention rate based on the interaction between the Vehicle Age and OnStar, in addition to the country of sale. This model is significant for any level and able to explain 80% of the variability of the retention rate: an excellent result.

Similarly, the analysis of the average invoice was performed, with a graphic representation first. The trend is that of an increase in the average bill over the years; however, OnStar raises the indicator only for vehicles with at least four years of life. Therefore, the long-term trend is positive, however in the analyzed period, the average invoice value remains lower for vehicles equipped with a black box, due to the values of the first three years.

After, a simple linear regression model was created; unfortunately, while significant, this model has a rather low explanatory power. This is because the linearity assumption is less strong than the retention rate forecast model.
Continuing by adding variables, we arrive at the complete model which in this case too uses the interaction between the age of the vehicle and the presence of OnStar, in addition to the country variable. This model achieves an R squared which is not extremely high, but certainly acceptable and is globally significant. Unfortunately, the coefficient for Spain is only significant for a 10% level; therefore, the model is less powerful in its forecasts than the one relating to the retention rate, but still usable.
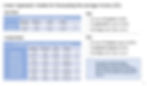
Finally, the analysis aims to provide suggestions to Opel management, basing on the conclusions of the models. Despite the limitations of the work, given by the absence of information regarding the vehicles’ models and a relatively short period of time, the OnStar experience was certainly positive. The system improves the retention rate, generating incremental turnover, in a business that presents many threats. Unfortunately, Opel no longer has this system available, as the brand was sold by General Motors to Groupe PSA, while OnStar was not included in the agreement. However, the merger between PSA and FCA, from which Stellantis was born, resulted in the availability of Mopar Connect system, FCA’s predictive maintenance tool, very similar to OnStar.
So, the group's goal for aftersales must be twofold: to extend the Mopar Connect system to all brands coming from the PSA group, saving various resources, while aiming to invest them in the development of a proprietary artificial intelligence system. That can give a competitive advantage for the future, thanks to the real-time monitoring potential, capable of increasing the perception of safety in end customers.
